Targeting in the Black Box: The Need to Reprioritize AI Explainability
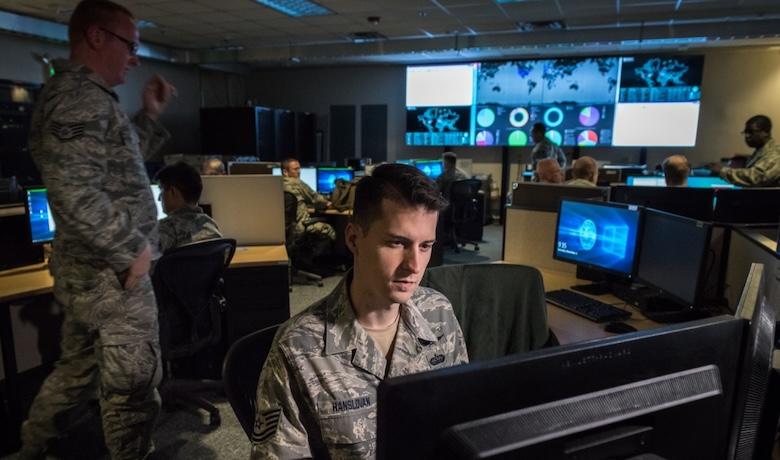
States around the world are racing to leverage the rapid evolution of artificial intelligence (AI) to their own military advantage. In the coming decades, AI-based systems are expected to revolutionize logistics, significantly alter targeting processes, and power increasingly complex and lethal autonomous weapons systems.
The armed conflicts in Gaza and Ukraine exemplify this trend. At the end of 2023, multiple outlets reported on Israel’s widespread use of an AI system to identify targets. Dubbed “Habsora,” or “the Gospel” in English, Israel Defense Forces (IDF) officials credited the system with the ability to increase the number of targetable sites in Gaza from 50 each year to over 100 each day. In Ukraine, quadcopters use an AI-powered autonomous tracking system to lock onto targets and deliver a lethal payload.
As this shift to integrate AI into new and existing weaponry advances, scholars and policymakers have sought to identify a workable framework by which such tools, especially in relationship to targeting and autonomy, can be effectively regulated. With so much uncertainty, new legal rules capable of garnering widespread State support remain elusive. In November 2023, the United States, along with several other countries, adopted the Political Declaration on Responsible Military Use of Artificial Intelligence and Autonomy. While not legally binding, the Declaration adopts a position upon which there is the greatest consensus among States, specifically that the “use of AI in armed conflict must be in accord with States’ obligations under international humanitarian law.”
Using this point of consensus, this post unpacks an underexamined challenge within the burgeoning literature surrounding military AI and targeting: How well do existing international humanitarian law (IHL) standards map onto the use of AI modeling systems whose very functionality remains mysterious to their users and designers?
The Black-Box Problem
Black box AI models can generally be understood as any model whose inputs and operations are opaque to users or other interested parties. As computer science scholars have set out, there are three basic kinds of opacity problems that can attach to AI systems: (1) opacity based on intentional concealment; (2) opacity due to technological illiteracy; and (3) opacity through cognitive mismatch.
Military AI systems are likely to suffer from all three. Information on how military AI targeting platforms function will undoubtedly and appropriately remain classified at the highest level. Further, even if such information was widely disseminated, few within the broader public would understand the technological underpinnings to a degree that would render the issue digestible as a matter of public policy.
However, the third type of opacity—a fundamental cognitive mismatch between the AI system and human information processing—poses the greatest challenge.
Artificial neural networks (ANNs), the kinds of systems responsible for most advances in AI right now, pose two major problems of understandability. The first flows from their tremendous complexity. ANNs require endless volumes of data and create millions, and sometimes billions, of parameters. The resulting prediction generated can involve millions of mathematical and computation operations, rendering the traceability from data to prediction functionally impossible. Perhaps more concerningly, the nature of an ANN’s architecture and operations is so cognitively dissimilar to humans that, as described by one scholar, there exists a fundamental “mismatch between [the model’s] nature and [human] understanding,” and as a result, the models “are not intelligible no matter how much knowledge we possess on mathematics, computation, or any other related science.” As such, not only are many models too complex to feasibly trace their parameters to their output, the means by which they come to those outcomes would render such traceability cognitively incoherent anyway.
The Role of Explanations in Law
Explanations play a crucial role in law. Domestically, law enforcement officers must articulate evidence and reasoning to justify an arrest, administrative agencies are expected to do the same in promulgating regulations, and judges author lengthy opinions to explain, legitimize, and shape legal rules.
Explanations are similarly crucial in the international legal space where obligations are drafted at a higher level of abstraction due to the large qualitative and quantitative differences in States’ economic, cultural, and legal circumstances. Within IHL, the legality of State (and sometimes individual) action frequently turns on the veracity of a proffered explanation.
As the use of AI-based targeting systems proliferates, government officials and those designing those systems need to carefully consider the crucial role of explainability embedded within the IHL principles of distinction, proportionality, and precautions in attack, that commanders employing such systems will confront.
Distinction
The principle and associated rules of distinction require that combatants differentiate between military targets and civilians through the exercise of reasonable judgment. It is in this area where AI targeting systems possess the greatest promise. Such systems, with their ability to ingest vast amounts of data to identify patterns, may generally perform more accurately (and certainly more quickly) than human operators. However, this theoretical advantage can quickly become more clouded beyond classic status-based targeting and in assessing the targeting of civilians directly participating in hostilities. The standard possesses such dynamism and fluidity that The Commander’s Handbook on the Law of Naval Operations suggests that “[t]here is no definition of direct part[icipation] in hostilities in international law” and, as such, “[c]ombatants in the field must make an honest determination” based on “all relevant available facts in the circumstances prevailing at the time.” Without an understanding of the basis underlying an AI targeting system’s outputs, the “reasonable judgment” and an “honest determination” regarding the facts of which a commander is unaware is difficult.
Proportionality
Proportionality poses greater challenges. The targeting rule of proportionality demands that the anticipated incidental harm to civilians be weighed against the expected concrete and direct military advantage gained by an attack. Essentially, the proportionality standard requires the balancing of two qualitatively different interests that are inherently subjective and context-dependent. AI systems may be able to calculate the potential harm to civilians with impressive accuracy, but assessing military advantage is not only more complex, it is inescapably dynamic as factual developments in the conflict and tactical and strategic goals and valuations are constantly in flux. In short, the advantage of a particular attack can vary depending on the broader strategic context, which is difficult for AI systems to fully grasp. Further, States have recognized that the type of subjectivity set out in engaging in proportionality’s balancing is one rooted in human judgment.
Canada, for example, has articulated that commanders must possess an “honest and reasonable expectation” as to the attack’s contribution. Perhaps even more strikingly, Germany has stated that “no abstract calculations [are] possible” in assessing military advantage; as damning a conclusion as to programmability as there ever was. The lack of explainability in black-box AI systems means that commanders may not be able to understand how the AI system has weighed these factors, leading to an unknown (and possibly unknowable) loss of control over the proportionality assessment. Perhaps worse, black-box proportionality assessments are impossible to assess in real-time and only marginally easier after-the-fact, likely resulting in a heavy veiling of the flaws endemic to attempting to program that which is potentially unprogrammable.
Precautions in Attack
Finally, the targeting law rules of precaution require that all feasible measures be taken to avoid or minimize harm to civilians. With respect to attacks, this includes verifying that targets are indeed military objectives and choosing means and methods of attack that minimize civilian harm. More generally, Article 57(1) of Additional Protocol I imposes upon State parties an affirmative obligation to take “constant care” in the “conduct of military operations” to spare civilians and civilian objects. The fulfillment of both requires an understanding of the basis of the targeting recommendation. It is difficult to verify a target of an attack if the commander is unaware of the information giving rise to the targetability determination and how it was weighed. Similarly, the “constant care” obligation has been understood to require such States to possess “situational awareness at all times” to avert civilian harm. Such situational awareness requires an understanding of not only the information giving rise to targetability, but also the military advantages perceived and the original assessment of civilian harm. It also demands awareness that the conditions are dynamic.
Reprioritizing Explainability
A significant role for human decision-makers has received major emphasis in efforts to regulate military AI applications and assuage public concern. Black-box AI models fundamentally compromise the integration of human operators by rendering such operators unaware of the context and reasoning influencing AI outputs.
Recognition of the importance of explainable AI (XAI) is not new. In 2016, the Defense Advanced Research Projects Agency (DARPA) launched an initiative asserting that XAI “will be essential if future warfighters are to understand, appropriately trust, and effectively manage an emerging generation of artificially intelligent machine partners.” NATO’s initial Artificial Intelligence Strategy identified “Explainability and Traceability” as a core principle that required military AI systems to “be appropriately understandable and transparent.”
Unfortunately, most recent efforts have moved away from XAI as a foundational principle guiding the development and use of AI in the military space. NATO’s Revised Artificial Intelligence Strategy, while recommitting itself to the core principles of its initial strategy and recognizing the emergence of more complex “frontier” models, offered no general or specific goals related to ensuring AI explainability. The U.S.-led Political Declaration on Responsible Military Use of Artificial Intelligence and Autonomy forgoes any reference to explainability or interpretability at all. Instead, it commits only that military AI systems be “developed with methodologies, data sources, design procedures and documentation that are transparent and auditable,” thus only addressing the constitutive parts of the model rather than the model itself.
The move away from XAI likely flows from a few sources. The launch of ChatGPT and other generative AI technologies whose operations remain mysterious has likely placed a premium on development first with explainability concerns to be addressed later. This predisposition might further be reinforced by what AI researchers call the “performance-explainability tradeoff.” This tradeoff articulates the belief that AI models that achieve better performance (often colloquially understood as “accuracy” among laypeople) tend to be less explainable. In short, the better a model performs the more opaque and uninterpretable its process and outputs and vice versa.
It is easy to understand the attractiveness of military AI applications. What commander wouldn’t want exponentially more targets available for consideration in pursuit of an operation, as Israel suggested has occurred in Gaza due to its AI targeting platforms? The proposition that the accuracy of such targeting identifications is greater than prior processes further makes the case. However, the rush to incorporate AI systems that neither designers nor operators understand will inexorably lead to catastrophe. While black-box models may (but also may not) offer greater performance than their more explainable counterparts, an inability to interpret their outputs leaves the humans entrusted with their use without the capacity to assess those outputs under the very human-oriented standards that IHL has established. That same inability to independently assess or understand an AI system’s output will, as DARPA stated, compromise a soldier’s ability to “appropriately trust and effectively manage” the AI platforms they are deploying.
***
Scott Sullivan is a Professor at the U.S. Military Academy at West Point with appointments at the Army Cyber Institute (ACI) and Department of Law and Philosophy. The views expressed here are those of the author’s alone and do not necessarily reflect that of the Department of Defense, the Department of the Army, ACI or any other entity or agency of the U.S. government.
Photo credit: Joseph Eddins